Timisoara_Med 2022, 2022(2), 4; doi:10.35995/tmj20220204
Review Article
Circulating microRNA-141 as a Biomarker for Prostate Cancer: A Systematic Review and Meta-Analysis
1
Department of Biochemistry & Pharmacology, Victor Babes University of Medicine and Pharmacy, 300041 Timisoara, Romania; nitusca.diana@umft.ro (D.N.); marcu.anca@umft.ro (A.M.); eseclaman@umft.ro (E.S.); ovidiu.sirbu@umft.ro (I.O.S.); cmarian@umft.ro (C.M.)
2
Department of Urology, Victor Babes University of Medicine and Pharmacy, 300041 Timisoara, Romania; razvan.bardan@umft.ro
3
Department of Functional Genomics, Proteomics and Experimental Pathology, The Oncology Institute “Prof. Dr. Ion Chiricuta”, 400015 Cluj-Napoca, Romania; ovidiu.balacescu@iocn.ro
4
Discipline of Public Health and Health Management, Department of Functional Sciences, Victor Babes University of Medicine and Pharmacy, 300041 Timisoara, Romania; bucur.adina@umft.ro
5
Center for Translational Research and Systems Medicine, “Victor Babes” University of Medicine and Pharmacy, 300041 Timisoara, Romania
*
Correspondence: sursoniu@umft.ro
How to cite: Nitusca, D.; Marcu, A.; Seclaman, E.; Bardan, R.; Sirbu, I.O.; Balacescu, O.; Bucur, A.I.; Ursoniu, S.; Marian, C. Circulating microRNA-141 as a biomarker for prostate cancer: A systematic review and meta-analysis. Timisoara Med. 2022, 2022(2), 4; doi:10.35995/tmj20220204.
Received: 22 November 2021 / Accepted: 20 August 2022 / Published: 23 September 2022
Abstract
:(1) Background and Objectives: Responding to the need for more optimized biomarkers for prostate cancer (PCa) diagnosis, a high number of studies have shown that microRNAs (miRNAs) could serve as novel, more reliable diagnostic tools. The usefulness of these miRNAs in assessing the presence of PCa is still under debate, given the discrepancies in their diagnostic performances. In this respect, hsa-miR-141 is among the miRNAs of particular interest, being very much investigated in PCa biology. (2) Material and Methods: Here, we provide a meta-analysis of miR-141 data published until May 2019, whose diagnostic accuracy was assessed using the Quality Assessment for Diagnostic Accuracy Studies-2 (QUADAS-2) tool, by analyzing several parameters including sensitivity, specificity, positive likelihood ratio (LR+), negative likelihood ratio (LR-), diagnostic odds ratio (DOR) and the area under the curve (AUC). The six studies that matched our selection and were included in our meta-analysis comprise 283 PCa patients, 114 healthy controls, with 84 patients suffering from benign prostate diseases. (3) Results and Conclusions: Our results indicate a very good diagnostic accuracy for miRNA-141 (0.78 sensitivity, 0.96 specificity, DOR of 89, LR+ of 21, LR- of 0.23 and AUC of 0.87), underlying its utility as a PCa diagnostic biomarker.
Keywords:
microRNA; prostate cancer; biomarkers; diagnostic value; miR-141Introduction
The 2020 cancer statistics showed that one out of five cancer diagnoses are prostate cancers (PCa), which makes PCa the most common malignancy in males worldwide, therefore explaining the substantial research effort made to better understand its molecular mechanisms [1,2]. However, in spite of the tremendous progress in PCa research achieved in the last decade, there is still a tremendous amount of inconsistency between studies aiming to propose novel and accurate diagnostic biomarkers [3,4].
PCa is currently diagnosed based on a combination of clinical symptoms, pathology investigations and a few biomarkers. The main problem of the current diagnostic strategies is their modest ability to detect early PCa and their disappointing lack of specificity, which might ultimately lead to overdiagnosis [5]. The most widely used PCa biomarker, prostate specific antigen (PSA), has been found increased in both malignant and non-malignant prostate-associated pathologies, such that the US Preventive Services Task Force warned against using PSA screening in men older than 75 years [6].
Of the wide spectrum of molecules screened as putative PCa biomarkers, the non-coding RNAs in general and miRNAs in particular stand out as of high interest, given their peculiar stability in basically all biological fluids [7,8,9,10].
MicroRNAs (miRNAs) are small, non-coding RNA molecules that regulate gene expression through base-pairing to complementary sequences in the 3′ untranslated region of target messenger RNAs, thus modulating gene expression either by cleavage of mRNA or by inhibiting protein translation [11,12,13,14]. MiRNAs have been found to play key regulator roles in a number of physiological and pathological processes, such as proliferation, differentiation, migration, apoptosis, and metabolism [12]. In addition, the expression level of an important number of miRNAs has been found to be dysregulated in all phases of PCa. Moreover, experimental and computational research studies investigating miRNAs and their target roles have categorized these small endogenous molecules as oncogenes, or tumor suppressor genes. Recent miRNA expression profiling analyses have also indicated the diagnostic potential of several miRNAs, underlying their potential as novel diagnostic biomarkers, but also as therapeutical targets [15].
Outlined as an oncogene, miR-141 has elicited a particular interest in the PCa research given its association with both the androgen-dependent and castration-resistant types of PCa [16,17]. However, when investigated as PCa biomarker, miR-141 has provided inconsistent results, due to differences between study designs, lack of uniformization between protocol methods, specimen types and cohort sizes. Herein, we conducted a meta-analysis to analyze the overall diagnostic value of miR-141 in PCa.
Materials and Methods
Search Strategy
The search has been devised by two team members (D.N. and A.M.), who interrogated PubMed, Embase and Web of Knowledge databases (up to May 2019) with the following combination of key words: (“microRNA 141” or “miRNA-141” or “miR-141”) and (“circulating” or “serum” or “plasma”) and (“prostate”) and (“diagnosis” or “ROC curve” or “sensitivity” or “specificity”). The investigators first performed an initial screening of titles and abstracts, followed by full-text article reading, and selection of those fit for the meta-analysis. Disagreements were solved via discussion with a third member of the team (CM).
Inclusion and Exclusion Criteria
Research articles inclusion criteria were: (1) involved circulating (serum/plasma/whole blood/PBMCs) miR-141 as diagnostic biomarker for PCa, (2) contained data regarding sensitivity, specificity, and AUC, (3) involved a statistical comparison between miRNA levels in PCa patients’ samples vs. healthy controls and/or other benign prostate-associated diseases, and (4) were published in English language.
Research articles exclusion criteria were: (1) non-original research papers, such as conference abstracts, letters, and reviews, (2) non-circulating miRNAs for PCa diagnostic, (3) circulating miRNAs other than serum, plasma or whole blood (e.g., urine, seminal fluid), and (4) insufficient data to match our interests.
Our study protocol was not prospectively registered.
Data Extraction
The following key data were extracted by two team members (D.N. and A.M.) from all the articles included in the meta-analysis: author; publication year; country; ethnicity of patients; number of PCa patients, healthy controls or with other (non-malignant) prostatic pathologies; type of specimen collected (serum/plasma/whole blood/peripheral blood mononuclear cells (PBMCs)); miRNA expression profiling method; sensitivity and specificity values; and AUC and 95% CI AUC.
Quality Assessment
QUADAS-2 was used by two investigators to assess the quality of each of the included research articles [18]. The four key domains, all assessed in terms of risk of bias, were: patient selection, index test, reference standard, flow and timing. The risk of bias is rated low if all signaling questions are “yes”; if any of the signaling questions are answered with “no”, a potential for bias exists. “Unclear” is used only with insufficient or improperly reported data to permit a judgment. Applicability concerns can be “high”, “low” or “unclear”. The results of the quality assessment were used to provide an evaluation of the overall quality of included studies and to investigate potential sources of heterogeneity.
Statistical Analysis
Statistical analyses were performed by Stata MP 15.1 (StataCorp LP, College Station, TX, USA) statistical software using metandi and midas commands. p < 0.05 denoted statistical significance. Between-study heterogeneity was evaluated with the I2 index and χ2 test. A value of I2 > 50 % and/or a p < 0.05 indicated heterogeneity. Overall accuracy of miR-141 assays, sensitivity and specificity were extracted from each study. True positives (TPs), true negatives (TNs), false positives (FPs) and false negatives (FNs) were calculated for each study.
The pooled results of positive and negative likelihood ratios (LR+ and LR−, respectively) and their 95 % confidence intervals (CI) were calculated using the random-effects model [19,20].
LR+ is the odds of a positive test result in a patient with PCa, whereas LR− is the odds of a positive result in an individual without the disease.
A pooled summary receiver operating characteristics (SROC) curve was constructed, and the AUC was calculated. The overall diagnostic accuracy of miR-141 can be estimated based on the aforementioned parameters. This plot includes an overall estimate of sensitivity and (1-specificity) across all studies, a 95% confidence region around this estimate and a 95% prediction region taking heterogeneity into account.
Forest plots of sensitivity and specificity estimates and their 95% CIs were constructed from every study [21,22].
Sensitivity analysis included: quantile plot of residual-based goodness-of fit; Chi-squared probability plot of squared Mahalanobis distances for assessment of the bivariate normality assumption; a spikeplot for checking for particularly influential observations using Cook’s distance; a scatter plot for checking for outliers using standardized predicted random effects (standardized level-2 residuals).
In order to detect the amount of heterogeneity between the studies regarding sample types, meta-regression analyses was conducted. Deeks’ funnel plot analysis was performed to explore the potential publication bias [23].
In order to estimate the post-test probabilities, clinical or patient-relevant utility of the diagnostic test was evaluated with Fagan’s nomogram [24].
Results
Characteristics of Included Studies
After using the search string of interest in the databases, we have identified 30 articles that were relevant. In total, 17 of these studies did not respect the inclusion criteria: five articles were describing new methods of quantifying gene expression, therefore not being focused on differences in expression levels of miR-141, three papers used other specimen types, such as cell lines, urinary extracellular vesicles, two were review papers, two showed a downregulation of miR-141 in the serum of PCa patients compared to healthy controls, while all the others showed upregulation of miR-141, one did not include miR-141 in the analysis, and four did not present comparative results of PCa patients vs. healthy controls, but with different stages of PCa (such as localized vs. metastatic). Furthermore, from the remaining 13 publications, seven articles were excluded due to insufficient data, and finally, we selected six articles [17,25,26,27,28,29] for our meta-analysis study.
The six remaining studies comprised an overall number of 481 participants, of which 283 patients, 114 healthy controls and 84 patients with benign prostate pathologies. The included studies were published between 2008 and 2018 and were conducted in China (two studies), USA, Poland, Russia and Ireland. Among the six studies, three studies used serum samples, one used plasma, one used PBMCs and one used whole blood. We have especially selected only blood-based analysis to reduce heterogeneity.
All studies have detected and quantified the expression level of miR-141 by quantitative real-time polymerase chain reaction, all showing up-regulation of miR-141 in PCa patients’ samples compared to healthy/benign controls.
Figure 1 summarizes the flow diagram of study selection, and Table 1 shows the characteristics of the six studies included in this meta-analysis.
Figure 1.
Flow-chart of the study selection process.
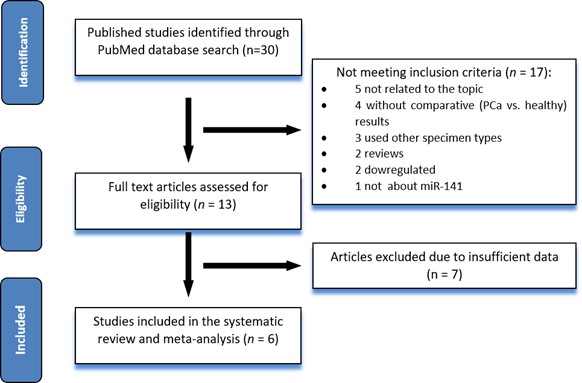
Table 1.
Characteristics of the six studies included in our meta-analysis.
Author | Year | Ethnicity | Country | Cases | Controls | Specimen | Endogenous Control | Sens. | Spec. | AUC |
---|---|---|---|---|---|---|---|---|---|---|
Guo et al. | 2018 | Asian | China | 72 | 34 | Serum | cel-miR-39 spike-in | 0.87 | 1 | 0.92 |
Mao et al. | 2018 | Asian | China | 43 | 57 BPH* | PBMCs | U6 | 0.80 | 0.48 | 0.62 |
Porzycki el al. | 2018 | Caucasian | Poland | 20 | 8 | Serum | U6 | 0.65 | 0.88 | 0.83 |
Mitchell et al. | 2008 | NA | USA | 25 | 25 | Serum | cel-miR-39 spike-in | 0.60 | 1 | 0.90 |
Osipov et al. | 2016 | Caucasian | Russia | 48 | 47 | plasma | miR-16, miR-101 | 0.56 | 1 | 0.80 |
Kelly et al. | 2015 | Caucasian | Ireland | 75 | 27 with benign disease | Whole blood | miR-16, miR-425 | 0.94 | NA | 0.65 |
Abbreviations: Sens. = sensivity; Spec. = specificity; AUC = area under the curve; BPH = benign prostatic hyperplasia; PBMCs = peripheral blood mononuclear cells.
Quality Assessment
There are two studies with a high risk of bias in the selection domain, while two are considered to be at an unknown risk of bias. Furthermore, on the index domain, there is another study with a high risk of bias. Two pther studies have an unknown risk of bias, thus a sensitivity analysis can find them in a possible scenario with a high risk of bias, according to the QUADAS-2 system (Table 2). The risk of bias and applicability concerns graph for the included studies is shown in Figure 2.
Table 2.
Summary of “risk of bias” and “applicability concerns” through judging each domain for each included study.
Table 2.
Summary of “risk of bias” and “applicability concerns” through judging each domain for each included study.
Study | Risk of Bias | Applicability Concerns | |||||
---|---|---|---|---|---|---|---|
Patient Selection | Index Test | Reference Standard | Flow and Timing | Patient Selection | Index Test | Reference Standard | |
Guo XJ/2018 | ![]() | ![]() | ![]() | ![]() | ![]() | ![]() | ![]() |
Mao ZJ/2018 | ![]() | ![]() | ![]() | ![]() | ![]() | ![]() | ![]() |
Porzycki P/2018 | ![]() | ![]() | ![]() | ![]() | ![]() | ![]() | ![]() |
Mitchell S/2008 | ![]() | ![]() | ![]() | ![]() | ![]() | ![]() | ![]() |
Osipov I/2016 | ![]() | ![]() | ![]() | ![]() | ![]() | ![]() | ![]() |
Kelly BD/2015 | ![]() | ![]() | ![]() | ![]() | ![]() | ![]() | ![]() |
Figure 2.
QUADAS-2 of the included studies.
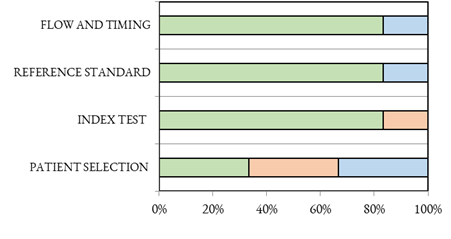
Diagnostic Accuracy of miR-141 in PCa
The test for the heterogeneity among the studies showed significant heterogeneity (I2 = 86.66 and 95.98% for sensitivity and specificity, respectively). For this reason, the random-effects model was used. Meta-analysis results showed the pooled sensitivity and pooled specificity of PCa by miR-141 were 0.78 (95% CI: 0.63–0.88) and 0.96 (95% CI: 0.28–1.00) in distinguishing PCa from control, respectively (Figure 3), with the area under receiver operating characteristic curve (AUC) of 0.87 (95% CI: 0.83–0.89) (Figure 4), thereby implying a relatively high diagnostic accuracy.
Figure 3.
Forest plots of sensitivity and specificity of mir-141 in distinguishing PCa from controls. Plots display diagnostic probabilities of included studies, and corresponding 95% confidence intervals. The rhombus represents the pooled estimates for specificity and sensitivity, respectively.
Figure 3.
Forest plots of sensitivity and specificity of mir-141 in distinguishing PCa from controls. Plots display diagnostic probabilities of included studies, and corresponding 95% confidence intervals. The rhombus represents the pooled estimates for specificity and sensitivity, respectively.
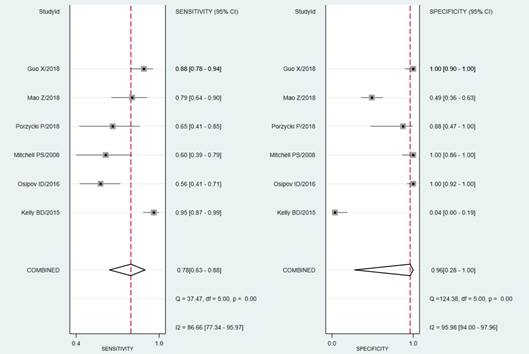
Figure 4.
Receiver operating characteristic graph of mir-141 in distinguishing PCa cases from controls, with 95% confidence region and 95% prediction region. Individual study estimates are represented as circles.
Figure 4.
Receiver operating characteristic graph of mir-141 in distinguishing PCa cases from controls, with 95% confidence region and 95% prediction region. Individual study estimates are represented as circles.
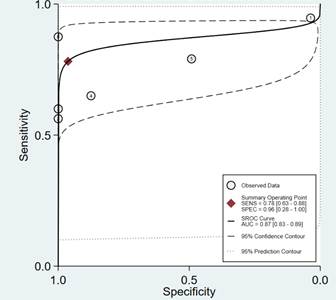
The average likelihood ratio of the positive and negative test result was calculated on the basis of the pooled estimates of sensitivity and specificity, and the results showed the LR+ and LR− of miR-141 in differentiating PCa from control patients were 21 (95% CI: 0.22–1844) and 0.23 (95% CI: 0.13–0.39), respectively. In addition, the mean DOR value was 89 (95% CI: 1.21–6547.37).
A meta-regression analysis was used to explore the potential source of heterogeneity in sensitivity and specificity based on sample types. Our analysis suggested that sample types (p < 0.001) might be the potential source of heterogeneity in specificity. Because of the small number of eligible studies, to fully elucidate the source of the heterogeneity, further studies would be needed.
Sensitivity Analysis and Publication Bias
Goodness-of-fit and bivariate normality analyses (Figure 5a,b) suggested that the random-effects bivariate model was robust for the calculation of the pooled estimates. No outlier was identified by influence analysis, and none appeared in the outlier detection plot (Figure 5c,d).
Figure 5.
Model-checking capabilities: graphical illustration of (a) residual-based goodness-of-fit, (b) bivariate normality, (c) influence analysis and (d) outlier detection.
Figure 5.
Model-checking capabilities: graphical illustration of (a) residual-based goodness-of-fit, (b) bivariate normality, (c) influence analysis and (d) outlier detection.
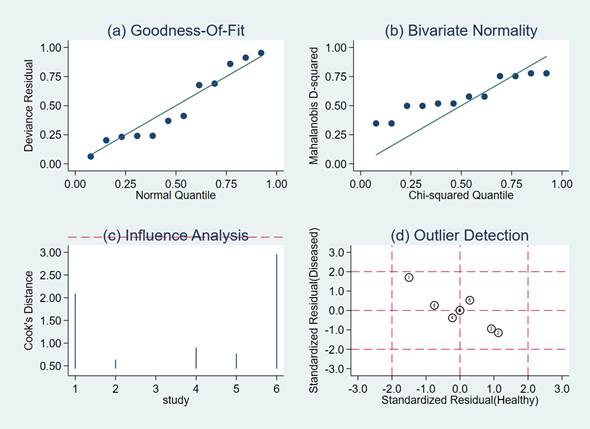
Fagan’s Nomogram for the likelihood ratio is shown in Figure 6.
Figure 6.
Fagan’s nomogram for estimating post-test probabilities.
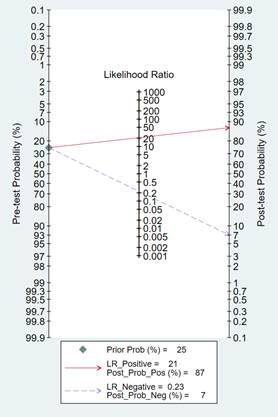
In later steps of this meta-analysis, we repeated the pooled sensitivity and specificity analysis with each of the studies removed individually. We found that the final results were similar to the initial result, reflecting stability and credibility of the results. As shown in Figure 7, Deeks funnel plot asymmetry test was not significant (p = 0.88), and there was no significant publication bias among the studies included in this analysis.
Figure 7.
Deeks’ linear regression test of funnel plot asymmetry.
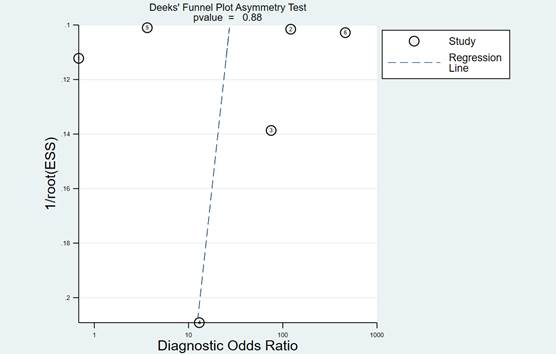
Discussion
PCa has gained a particular interest in research and clinics, because it is nowadays one of the most over-diagnosed types of cancer in men (mostly due to the lack of PSA specificity) [30,31]. Novel biomarkers, such as miRNAs are being investigated for their potential diagnostic accuracy. Many studies that investigated the potential role of miRNAs as biomarkers in PCa arose by measuring the differences in their expression level in PCa patients versus healthy controls (or other types of benign prostatic diseases) [32,33,34].
The main goal of this meta-analysis was to investigate the true diagnostic value for miR-141 in PCa, by analyzing the studies published until 2018 regarding this particular miRNA. We have mainly focused on predicting the diagnostic accuracy of miR-141 for PCa, by pooling together a total number of six studies that investigated the differences in expression levels of circulating miR-141 from PCa patients’ samples (serum, plasma, whole blood or PBMCs) compared to healthy controls, or patients with BPH. The pooled values for sensitivity, specificity, and AUC (0.78, 0.96 and 0.87, respectively) prove that miR-141 has a relatively high overall diagnostic accuracy for PCa, and it holds great promise for the future diagnosis of this malignancy. The overall accuracy of miR-141 in PCa diagnosis is surprisingly high, as demonstrated by the DOR value of 89.
MiR-141 is an oncogenic type of miRNA, therefore its expression level is higher in malignant tissues compared to healthy prostatic tissues and benign prostatic hyperplasia (BPH) [35,36]. In addition, transfecting pre-miR-141 into Lymph Node Carcinoma of the Prostate cells increased cells proliferation, while anti-miR-141 transfection suppressed their growth. Interestingly, miR-141 is expressed significantly higher in androgen receptor (AR) positive xenografts compared to negative AR xenografts [37].
Brase et al. described up-regulation of circulating miR-141 in patients with metastases, suggesting it could differentiate metastatic PCa from healthy subjects [38]. Interestingly, Mitchell et al. showed that miR-141 is highly expressed in prostate epithelial cells and absent in stromal cells [17].
MiR-141 was shown to be an ideal candidate as a circulating biomarker, due to its distribution in plasma, serum, whole blood, or PBMCs. The liquid biopsy technique is one of the most minimally invasive types of analysis, and it is of high interest for miRNA biomarker research, given the important amounts of circulating miRNAs specific to their area of origin that can be found in biological fluids [39].
This is the first meta-analysis to ever assess the true diagnostic value of miR-141 in PCa in similar sample specimens (blood-based), without the inclusion of other biological samples (tissue biopsy, urine, etc.), in order to reduce heterogeneity as much as possible. We strongly believe that this might represent a major strength of our research, as future minimally invasive biomarkers for these types of malignancies might be screened rather from blood specimens or other circulating samples, than from tissue samples that involve invasive procedures. Nevertheless, there still remains some degree of heterogeneity among sample types that could induce bias, as there might be differences in miRNA expression between acellular samples such as serum and plasma samples, and samples containing blood cells. This aspect will need to be further addressed by future studies.
Nonetheless, our meta-analysis encompassed some limitations as well, which are worth acknowledging. First, the control group was not the same between the studies, as we have retrieved articles with either healthy or with benign disease controls (that might have by itself different values for miR-141), which might introduce a selection bias. Secondly, we found it quite challenging to find studies with similar research designs, methodology and sample specimens, and therefore the search has been considerably narrowed down in order to include articles as similar as possible. Thirdly, the selection of patients and/or the patient flow might have introduced bias, although we cannot know for sure, as patients’ clinical information was quite scarce in some of the articles. A threshold value was used, at least in some articles, while others lacked this information, so we decided to remove that data for all. Moreover, the wide confidence intervals are due to the small number of included studies. Last but not least, by performing this meta-analysis, we have observed that there is a lack of uniformization and standardization of research protocols and methodologies, with considerable discrepancies between studies with similar scopes, which would need further discussion in future research.
Conclusions
The results of this meta-analysis study suggest that miR-141 might represent a potential biomarker for PCa diagnosis. However, future large-scale studies are needed to definitely evaluate the reliability of miR-141 as biomarker in PCa diagnosis.
Author Contributions
Conceptualization, C.M. and S.U.; methodology, A.B.; software, S.U.; validation, C.M., E.S. and I.O.S; formal analysis, O.B.; investigation, A.M.; resources, C.M.; data curation, R.B.; writing—original draft preparation, D.N.; writing—Review and Editing, D.N.; visualization, A.M.; supervision, C.M.; project administration, S.U.; funding acquisition, C.M. All authors have read and agreed to the published version of the manuscript.
Funding
This research was funded by UEFISCDI grant no. PN-III-P4-IDPCE-2016-0371.
Conflicts of Interest
The authors declare no conflict of interest.
References
- Siegel, R.L.; Miller, K.D.; Jemal, A. Cancer statistics. CA Cancer J. Clin. 2016, 66, 7–30. [Google Scholar] [CrossRef] [PubMed]
- Siegel, R.L.; Miller, K.D.; Jemal, A. Cancer statistics. CA Cancer J. Clin. 2018, 68, 7–30. [Google Scholar] [CrossRef] [PubMed]
- Wyatt, A.W.; Mo, F.; Wang, Y.; Collins, C.C. The diverse heterogeneity of molecular alterations in prostate cancer identified through next-generation sequencing. Asian J. Androl. 2013, 15, 301–308. [Google Scholar] [CrossRef] [PubMed]
- Mitchell, T.; Neal, D.E. The genomic evolution of human prostate cancer. Br. J. Cancer 2015, 113, 193–198. [Google Scholar] [CrossRef] [PubMed]
- Welch, H.G.; Albertsen, P.C. Prostate cancer diagnosis and treatment after the introduction of prostate-specific antigen screening: 1986-2005. J. Natl. Cancer Inst. 2009, 101, 1325–1329. [Google Scholar] [CrossRef] [PubMed]
- Agency for Healthcare Research and Quality: Screening for Prostate Cancer. Available online: http://www.ahrq.gov/clinic/uspstf/uspsprca.htm (accessed on 12 March 2022).
- Wang, G.; Chan, E.S.; Kwan, B.C.; Li, P.K.; Yip, S.K.; Szeto, C.C.; Ng, C.F. Expression of microRNAs in the urine of patients with bladder cancer. Clin. Genitourin. Cancer 2012, 10, 106–113. [Google Scholar] [CrossRef]
- Cifuentes, D.; Xue, H.; Taylor, D.W.; Patnode, H.; Mishima, Y.; Cheloufi, S.; Ma, E.; Mane, S.; Hannon, G.J.; Lawson, N.D.; et al. A novel miRNA processing pathway independent of Dicer requires Argonaute2 catalytic activity. Science 2010, 328, 1694–1698. [Google Scholar] [CrossRef]
- Watanabe, Y.; Tomita, M.; Kanai, A. Computational methods for microRNA target prediction. Methods Enzymol. 2007, 427, 65–86. [Google Scholar]
- Saito, T.; Saetrom, P. MicroRNAs--targeting and target prediction. New Biotechnol. 2010, 27, 243–249. [Google Scholar] [CrossRef]
- Bartel, D.P. MicroRNAs: Genomics, biogenesis, mechanism, and function. Cell 2004, 116, 281–297. [Google Scholar] [CrossRef]
- Jansson, M.D.; Lund, A.H. MicroRNA and cancer. Mol. Oncol. 2012, 6, 590–610. [Google Scholar] [CrossRef] [PubMed]
- Hata, A.; Lieberman, J. Dysregulation of microRNA biogenesis and gene silencing in cancer. Sci. Signal. 2015, 8, re3. [Google Scholar] [CrossRef]
- Wahid, F.; Shehzad, A.; Khan, T.; Kim, Y.Y. MicroRNAs: Synthesis, mechanism, function, and recent clinical trials. Biochim. Biophys. Acta 2010, 1803, 1231–1243. [Google Scholar] [CrossRef] [PubMed]
- Vanacore, D.; Boccellino, M.; Rossetti, S.; Cavaliere, C.; D’Aniello, C.; Di Franco, R.; Romano, F.J.; Montanari, M.; Mantia, E.L.; et al. Micrornas in prostate cancer: An overview. Oncotarget 2017, 8, 50240–50251. [Google Scholar] [CrossRef] [PubMed]
- Zhang, H.-L.; Qin, X.-J.; Cao, D.-L.; Zhu, Y.; Yao, X.-D.; Zhang, S.-L.; Dai, B.; Ye, D.-W. An elevated serum miR-141 level in patients with bone-metastatic prostate cancer is correlated with more bone lesions. Asian J. Androl. 2013, 15, 231–235. [Google Scholar] [CrossRef]
- Mitchell, P.S.; Parkin, R.K.; Kroh, E.M.; Fritz, B.R.; Wyman, S.K.; Pogosova-Agadjanyan, E.L.; Peterson, A.; Noteboom, J.; O’Briant, K.C.; Allen, A.; et al. Circulating microRNAs as stable blood-based markers for cancer detection. Proc. Natl. Acad. Sci. USA 2008, 105, 10513–10518. [Google Scholar] [CrossRef]
- Whiting, P.F.; Rutjes, A.W.S.; Westwood, M.E.; Mallett, S.; Deeks, J.J.; Reitsma, J.B.; Leeflang, M.M.G.; Sterne, J.A.C.; Bossuyt, P.M.M.; QUADAS-2 Group. QUADAS-2: A revised tool for the quality assessment of diagnostic accuracy studies. Ann. Intern. Med. 2011, 155, 529–536. [Google Scholar] [CrossRef]
- Devillé, W.L.; Buntinx, F.; Bouter, L.M.; Montori, V.M.; de Vet, H.C.W.; van der Windt, D.A.W.M.; Bezemer, P.D. Conducting systematic reviews of diagnostic studies: Didactic guidelines. BMC Med. Res. Methodol. 2002, 2, 9. [Google Scholar] [CrossRef]
- DerSimonian, R.; Laird, N. Meta-analysis in clinical trials. Control Clin Trials 1986, 7, 177–188. [Google Scholar] [CrossRef]
- Dinnes, J.; Deeks, J.; Kirby, J.; Roderick, P. A methodological review of how heterogeneity has been examined in systematic reviews of diagnostic test accuracy. Health Technol. Assess. 2005, 9, 1–113. [Google Scholar] [CrossRef]
- Higgins, J.P.T.; Thompson, S.G.; Deeks, J.J.; Altman, D.G. Measuring inconsistency in meta-analyses. BMJ 2003, 327, 557–560. [Google Scholar] [CrossRef] [PubMed]
- Deeks, J.J.; Macaskill, P.; Irwig, L. The performance of tests of publication bias and other sample size effects in systematic reviews of diagnostic test accuracy was assessed. J. Clin. Epidemiol. 2005, 58, 882–893. [Google Scholar] [CrossRef] [PubMed]
- Fagan, T.J. Letter: Nomogram for Bayes theorem. N. Engl. J. Med. 1975, 293, 257. [Google Scholar] [PubMed]
- Guo, X.; Han, T.; Hu, P.; Guo, X.; Zhu, C.; Wang, Y.; Chang, S. Five microRNAs in serum as potential biomarkers for prostate cancer risk assessment and therapeutic intervention. Int. Urol. Nephrol. 2018, 50, 2193–2200. [Google Scholar] [CrossRef]
- Porzycki, P.; Ciszkowicz, E.; Semik, M.; Tyrka, M. Combination of three miRNA (miR-141, miR-21, and miR-375) as potential diagnostic tool for prostate cancer recognition. Int. Urol. Nephrol. 2018, 50, 1619–1626. [Google Scholar] [CrossRef] [PubMed]
- Osipov, I.D.; Zaporozhchenko, I.A.; Bondar, A.A.; Zaripov, M.M.; Voytsitskiy, V.E.; Vlassov, V.V.; Laktionov, P.P.; Morozkin, E.S. Cell-Free miRNA-141 and miRNA-205 as Prostate Cancer Biomarkers. Adv. Exp. Med. Biol. 2016, 924, 9–12. [Google Scholar]
- Kelly, B.D.; Miller, N.; Sweeney, K.J.; Durkan, G.C.; Rogers, E.; Walsh, K.; Kerin, M.J. A Circulating MicroRNA Signature as a Biomarker for Prostate Cancer in a High Risk Group. J. Clin. Med. 2015, 4, 1369–1379. [Google Scholar] [CrossRef]
- Mao, Z.; Ji, A.; Yang, K.; He, W.; Hu, Y.; Zhang, Q.; Zhang, D.; Xie, L. Diagnostic performance of PCA3 and hK2 in combination with serum PSA for prostate cancer. Medicine (Baltimore) 2018, 97, e12806. [Google Scholar] [CrossRef]
- Misawa, A.; Takayama, K.I.; Inoue, S. Long non-coding RNAs and prostate cancer. Cancer Sci. 2017, 108, 2107–2114. [Google Scholar] [CrossRef]
- Kurul, N.O.; Ates, F.; Yilmaz, I.; Narli, G.; Yesildal, C.; Senkul, T. The association of let-7c, miR-21, miR-145, miR-182, and miR-221 with clinicopathologic parameters of prostate cancer in patients diagnosed with low-risk disease. Prostate 2019, 79, 1125–1132. [Google Scholar] [CrossRef]
- Haldrup, C.; Kosaka, N.; Ochiya, T.; Borre, M.; Høyer, S.; Orntoft, T.F.; Sørensen, K.D. Profiling of circulating microRNAs for prostate cancer biomarker discovery. Drug Deliv. Transl. Res. 2014, 4, 19–30. [Google Scholar] [CrossRef] [PubMed]
- Watahiki, A.; Macfarlane, R.J.; Gleave, M.E.; Crea, F.; Wang, Y.; Helgason, C.D.; Chi, K.N. Plasma miRNAs as biomarkers to identify patients with castration-resistant metastatic prostate cancer. Int. J. Mol. Sci. 2013, 14, 7757–7770. [Google Scholar] [CrossRef] [PubMed]
- Selth, L.A.; Townley, S.L.; Bert, A.G.; Stricker, P.D.; Sutherland, P.D.; Horvath, L.G.; Goodall, G.J.; Butler, L.M.; Tilley, W.D. Circulating microRNAs predict biochemical recurrence in prostate cancer patients. Br. J. Cancer 2013, 109, 641–650. [Google Scholar] [CrossRef]
- Richardsen, E.; Andersen, S.; Melbø-Jørgensen, C.; Rakaee, M.; Ness, N.; Al-Saad, S.; Nordby, Y.; Pedersen, M.I.; Dønnem, T.; Bremnes, R.M.; et al. MicroRNA 141 is associated to outcome and aggressive tumor characteristics in prostate cancer. Sci. Rep. 2019, 9, 386. [Google Scholar] [CrossRef]
- Hao, X.-K.; Li, Z.; Ma, Y.-Y.; Wang, J.; Zeng, X.-F.; Li, R.; Kang, W. Exosomal microRNA-141 is upregulated in the serum of prostate cancer patients. Oncol. Targets Ther. 2015, 9, 139–148. [Google Scholar] [CrossRef]
- Waltering, K.K.; Porkka, K.P.; Jalava, S.E.; Urbanucci, A.; Kohonen, P.J.; Latonen, L.M.; Kallioniemi, O.P.; Jenster, G.; Visakorpi, T. Androgen regulation of micro-RNAs in prostate cancer. Prostate 2011, 71, 604–614. [Google Scholar] [CrossRef] [PubMed]
- Brase, J.C.; Johannes, M.; Schlomm, T.; Fälth, M.; Haese, A.; Steuber, T.; Beißbarth, T.; Kuner, R.; Sültmann, H. Circulating miRNAs are correlated with tumor progression in prostate cancer. Int. J. Cancer 2011, 128, 608–616. [Google Scholar] [CrossRef]
- Palmirotta, R.; Lovero, D.; Cafforio, P.; Felici, C.; Mannavola, F.; Pellè, E.; Quaresmini, D.; Tucci, M.; Silvestris, F. Liquid biopsy of cancer: A multimodal diagnostic tool in clinical oncology. Ther. Adv. Med. Oncol. 2018, 10. [Google Scholar] [CrossRef]
© 2022 Copyright by the authors. Licensed as an open access article using a CC BY 4.0 license